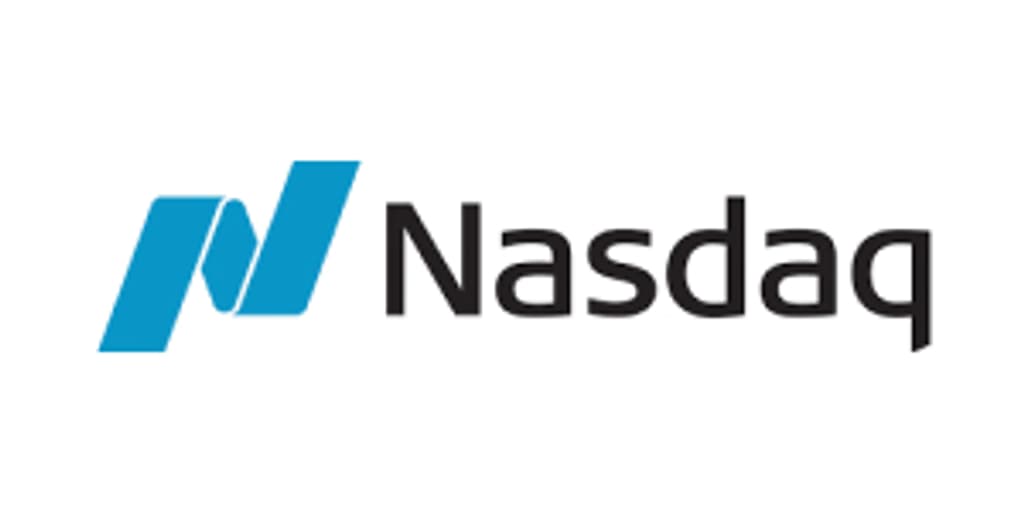
How Artificial Intelligence Transforms Accounts Receivable and Makes it More Human
- 11 min read
Artificial intelligence and machine learning in accounts receivable is making it more efficient and simultaneously more human.
In this blog, learn what ML in AR is, and how AI is actually critical to delivering more human accounts receivable experiences.
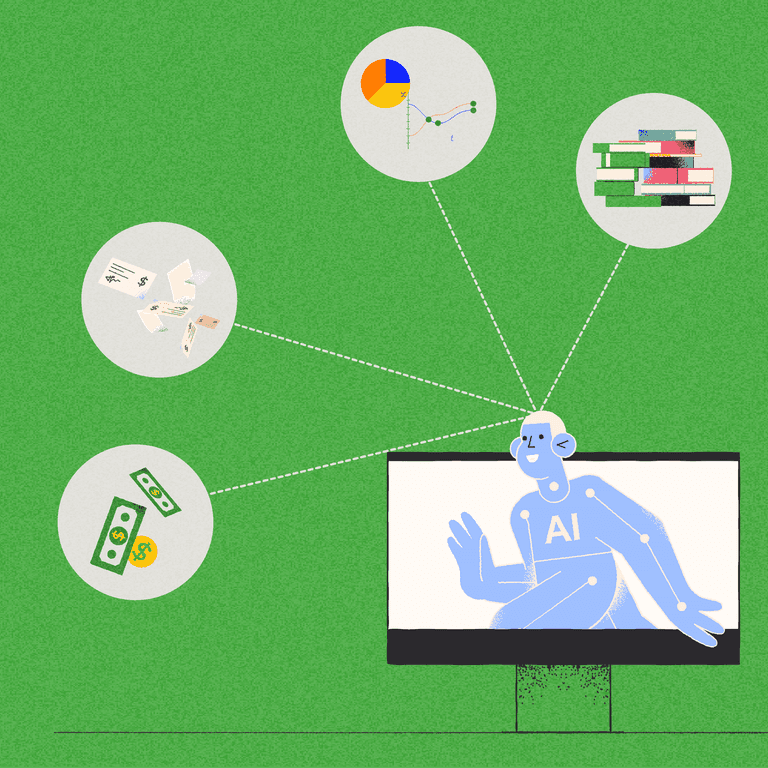
From trading algorithms to robotic process automation (RPA) in trade settlement, institutional finance firms have long experimented with and relied on artificial intelligence (AI) to automate tasks.
As technology has improved, these AI applications are trickling down to more financial functions, most notably accounts receivable (AR). While several AI applications in AR are theoretical, its presence is currently significant enough for enterprises to evaluate it as a part of their digital transformation initiatives.
In this article, we look at how, contrary to popular perception, AI is making accounts receivable more human, along with the following:
- What machine learning is in accounts receivable
- How machine learning transforms accounts receivable
- 5 common challenges of deploying AI in finance and how to overcome them
- Why AI adoption is critical for a more human accounts receivable workflow
- What the future holds for AI in accounts receivable
What is machine learning in accounts receivable?
Machine learning (ML) is a process where algorithms figure out their growth path and evolution without significant human input. Much like a human being assimilates information when learning a new subject, machine learning algorithms ingest, analyze, and apply insights from data to build their knowledge.
Most ML processes have significant human input during the initial training phase. In this stage, humans feed and routinely correct the machine's conclusions. As it moves onto the right path, humans flag exceptional cases that the algorithm cannot identify.
Machine learning in accounts receivable has several current and potential applications such as:
- Executing cash application processes.
- Projecting cash flow and working capital levels from recent invoice payments.
- Predicting the probability of a customer paying an invoice on time—a theoretical application.
- Estimating the probability of a customer dispute based on prior interactions and payment data—another theoretical application.
The 3 types of machine learning
There are 3 types of machine learning you must be aware of:
- Supervised learning—In this model, humans annotate and label data, guiding the algorithm as it takes its first steps. If the algorithm's output is inaccurate, humans adjust the frequency and weight of some datasets to guide it, instead of increasing the size of the dataset.
- Unsupervised learning—Human intervention is minimal in this model. Supervisors feed the algorithm a vast amount of data and let the machine figure out patterns and other trends to arrive at conclusions. If the output is incorrect, supervisors increase the size of the input dataset.
- Semi-supervised or hybrid learning—A combination of the previous two models, humans initially annotate input data. Following that, they feed the machine raw data, helping it figure out patterns in the input.
Examples of AI and ML applications in finance
Here are a few examples of AI and ML applications in finance:
- Trading algorithms
- Cash application in accounts receivable
- Cash flow projection based on paid invoices
- Credit evaluation
- Automating customer interactions
- Offer underwriting policy inputs
- Trading risk management
Firms like Kensho and Alphasense are major AI application developers targeting institutional financial firms. Versapay acquired AI developer Dade Systems in 2022 to power AR workflows with ML insights.
Machine learning and the digital transformation of accounts receivable
Accounts receivable teams have long been a part of the back office despite their customer-facing constituents. In fact, we’d argue that a direct link exists between customer experience (CX) and the invoice-to-cash process. After all, AR maintains frequent touchpoints with customers, often performing duties including handling customer payments, addressing invoice disputes, and managing collections outreach.
Digital transformation is gathering speed in accounts receivable, and integrating machine learning into routine workflows is the next logical step. AI-assisted accounts receivable is critical to minimizing the AR Disconnect—a term that refers to the misalignment and widening communication gap between customers and AR teams, creating poor customer experiences and easily-avoidable disputes.
The risks of not adopting AI in accounts receivable
While AI might not play a central role in AR workflows currently, firms that adopt ML-driven transformation will position themselves ideally for the future. The opportunity costs of losing out on efficiency gains will eventually catch up to the non-adopters, harming their business.
Research conducted by APQC indicates that the median cost of processing an invoice is $2.80. However, companies in the 75th percentile spend $6.00 to process an invoice—a number that adds up quickly when processing hundreds of thousands of them.
While automation has reduced the cost of invoice processing considerably (down from a median of $3.94 in 2018 according to APQC,) AI will lower these costs even more. Currently, the cost of processing 10,000 invoices every year is ($2.8 * 10000) $28,000. If AI increases efficiency by 30% (comparable to the efficiency gains automation has delivered since 2018), invoice processing costs reduce by $8,400 per 10,000 invoices.
That’s a significant gain whichever way you look at it.
The benefits of adopting AI in accounts receivable
There are many organization-wide benefits to adopting AI within your accounts receivable workflows. Here are some of the biggest ones:
- More value-added work—Your AR team spends more time solving complex customer disputes such as reconciling line item discrepancies since AI handles the simple ones like payment application or remedying item or service quantity errors. The result is a more human accounts receivable workflow that enhances customer relationships, since AR teams can devote themselves more to fostering and maintaining them.
- Greater efficiency—AI can instantly apply cash to open invoices, for instance, and save your AR team the hassle of chasing receipts and remittance advice and verifying supporting documentation—not to mention having to manually pull it from various channels. The result is more time for root cause analysis that boosts cash flow by removing impediments to it.
—
With AI-powered cash application, you can get way more done with a much smaller team. Learn how in this insight-packed on-demand webinar.
5 common challenges when deploying AI in finance
Deploying artificial intelligence in financial functions has its challenges. Here are the five most common ones and how you can overcome them.
1. Opaque nature of AI leads to drawing wrong conclusions
AI can be an opaque box, especially when using unsupervised learning models. A side effect of this situation is the model generates wildly unpredictable results at unexpected times. Even supervised models fail to account for Black Swan (highly improbable) events.
Thoroughly testing output and feeding the algorithm a steady diet of diverse data is the best solution to this problem.
2. Poor data organization
Take the time to prepare and validate your datasets before feeding them to your AI engine. Generating synthetic data that emulates existing real-world data is a good way of supercharging the AI learning process.
AI depends heavily on the data you feed it. Feed it biased data, and you'll receive biased results. For example, let's assume one of your best customers has run into temporary difficulties and has been paying invoices late over the past year.
A human being can immediately understand the context and predict that the situation is an exception. However, if you feed AI just the past year's data, it will conclude this customer is problematic and deserves stringent credit terms. As a result, if left unsupervised, your AI might implement a policy that damages your relationship with a loyal customer.
3. Lack of algorithm maintenance
A machine learning algorithm might be self-learning, but you must check its assumptions periodically. Fail to do this, and you'll risk the machine reaching conclusions that are highly incompatible with your business.
Schedule periodic maintenance and examinations as your AI program ramps up. This workflow will prevent any surprises.
4. Lack of qualified data science personnel
ML algorithms can automate several processes in your finance workflows. However, they rely on high-quality data, and you need qualified data science personnel to monitor everything from data governance to sourcing.
Data science skills are tough to train since human beings are prone to several psychological biases. For instance, present a business intelligence dashboard to a non-technical user, and you'll likely receive a bunch of incorrect conclusions. Data science professionals are at a premium right now, given the rapid pace at which companies have adopted data-centric workflows.
Offering data access to everyone (data democratization) in your organization will generate insights. However, you cannot guarantee the lack of biases unqualified data analysis professionals will bring to their conclusions. In the long run, you might chase the wrong goals and compound opportunity costs.
5. Improper edge case monitoring
ML algorithms often stumble on edge cases—situations that are exceptions because they are improbable or do not occur with high frequency. For example, 95% of your customers earning revenues greater than 10 million might pay their invoices within 60 days. However, 5% of them pay within 20 days.
This 5% of customers are the edge case. When learning, an ML algorithm cannot distinguish between a normal and an edge case. You need a data science professional to educate the algorithm and modify datasets to include edge cases in the right frequency. Include too many edge cases, and the algorithm will believe the edge case is normal.
Why AI adoption is critical for more human accounts receivable
Collaboration between customers and AR teamsis critical to bridging the AR Disconnect. Any digital transformation initiative in AR must prioritize this goal instead of merely automating AR workflows. Collaboration aligns your teams with your customers' goals and boosts CX.
At its core, great CX boils down to presenting a human face to your customers. Here's how AI makes your accounts receivable more human:
- AI boosts value-added AR work — Your AR team can focus on solving complex customer issues that need empathy and involvement thanks to document-heavy—and time-consuming—manual tasks like cash application handled by AI.
- AI boosts personalization—AI can alert you to changing patterns in customer behavior, allowing your AR team to proactively inquire about and solve any issues before they snowball—or in some cases, truly materialize. This hypothetical scenario captures the personalized experience AI and human beings can deliver when aligned.
- AI offers predictive insights—An ML engine could predict which invoices are likely to become overdue, giving your AR team a chance to inform cash flow forecasts in advance. The result is robust financial projections that help you create accurate cash projections and build more efficiency in financial workflows.
What the future holds for AI in accounts receivable
While cash application is AI's most prominent use case in accounts receivable right now, future applications such as predictive analytics in invoice payments, cash projections, and customer credit analysis are in the works.
AI can transform AR into a leading force for CX—something every organization must leverage if it wishes to future-proof its business. Take our six minute AR transformation assessment to receive your customized transformation roadmap now.
About the author

Vivek Shankar
Vivek Shankar specializes in content for fintech and financial services companies. He has a Bachelor's degree in Mechanical Engineering from Ohio State University and previously worked in the financial services sector for JP Morgan Chase, Royal Bank of Scotland, and Freddie Mac. Vivek also covers the institutional FX markets for trade publications eForex and FX Algo News. Check out his LinkedIn profile.